One of the biggest challenges facing banks today is how to effectively analyse and use the transaction data that they process in their systems. Time and time again, discussion has centred around the goldmine that is consumer banking data and its many use cases. But in reality, many retail banks struggle with data categorisation so that it can be used to produce real-time insights and actionable recommendations.
There are many layers to personalisation in digital banking, one of the first and most crucial layers is the categorisation of data. It is easy to get carried away with the UX design of an in-app widget or the look of a cross-sell advertisement. But if the data that is being used is not properly analysed and categorised the result will be financial wellbeing programmes or simple marketing use cases that lack the insight and intelligence to drive real engagement and change amongst consumers.
That is why categorisation of data, while only a small part of the personalisation pie, plays a pivotal role in driving customer engagement in digital banking.
What does categorisation of data mean?
In the context of banking specifically, categorisation of data refers to the organisation of transaction data based on pre-defined criteria, such as narratives, transaction types, merchant category codes (MCC) and other meta data, in order to facilitate the correct definition and grouping of transactions and form the basis for hyper-personalisation across banking channels.
Why is data categorisation important?
Transaction data contains some of the most useful information for banks to understand their customers. It provides incredible insight into how people live their lives, the things they like to do, the places they like to eat at, the countries they travel to, the items they regularly purchase, their sources of income and more. Categorisation makes data smarter, and more useful. It enables banks and their customers to get a clear and organised view of their everyday financial activities. By applying categories and other enrichments to transactions, it becomes easier to track, analyse and predict past and future income and expenditure, and more generally behaviours.
How does the Moneythor engine categorise transaction data?
To provide richer, actionable data to generate intelligent money management & loyalty features as well as personalised insights, the Moneythor engine performs transaction categorisation & enrichment in real-time and at scale across all types of accounts, cards, and e-wallets.
1. Raw Data
Moneythor’s high-performance engine consumes streaming or batched data sets of customer information across all their assets and liabilities such as accounts, cards, or digital wallets, as well as other banks’ data through Open Banking.
2. Categorised Data
From the raw data provided, the Moneythor engine looks for patterns and similar transactions to automatically assign the most accurate categories and enrichment strategies. The engine uses multi-lingual text analysis, rules based on regular expressions and priority levels, machine learning, external services where applicable and learning from individual customers’ choices as usage increases. Here is what is happening under the hood when categorisation takes place:
For transactions that cannot be automatically categorised or in the case that customised categories are preferred, categories can also be manually assigned in a self-service way by customers, triggering an automated learning process by the engine.
Each bank can also choose the most appropriate list of default categories to offer its customers, which can be customised depending on local needs and language preferences.
3. Augmented Data
Once categorised, the Moneythor engine can augment data further through transaction cleansing, adding merchant logos, detecting recurring patterns, bills and subscriptions, developing forecasts and more. From there, this categorised and augmented data is used to trigger personalised experiences across digital banking channels.
What if a customer wants to personalise categories?
From time to time, the way customers view their transactions may be different to how their bank would classify them.
That is why empowering customers with user-friendly opportunities to provide their feedback to the automated categorisation process is a must when presenting them with this information in digital banking channels.
Customers can add their own categories on the fly, potentially mix them with notes and hence truly personalise their experience. Over time, the engine learns such customer preferences and automatically apply them to subsequent transactions.
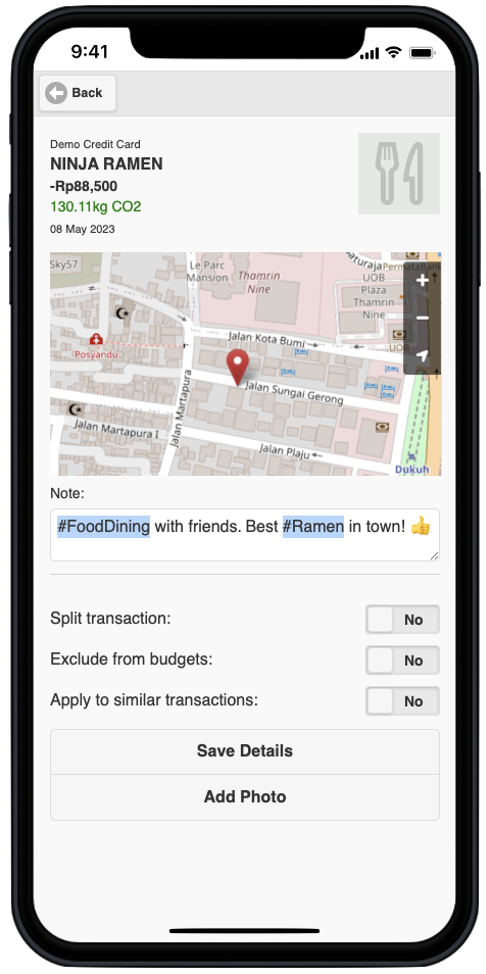
Bad data in, leads to bad data and poor experiences out. Categorisation and augmentation of data are critical for the successful implementation of personalised digital banking experiences. At Moneythor, we pride ourselves on providing our banking and fintech clients with advanced categorisation & enrichment capabilities, and a truly flexible and scalable engine.
Get in touch if you would like to learn more about how you could make more of your raw transaction data.